Why FICO Scores Aren’t Enough For Small Business Lending
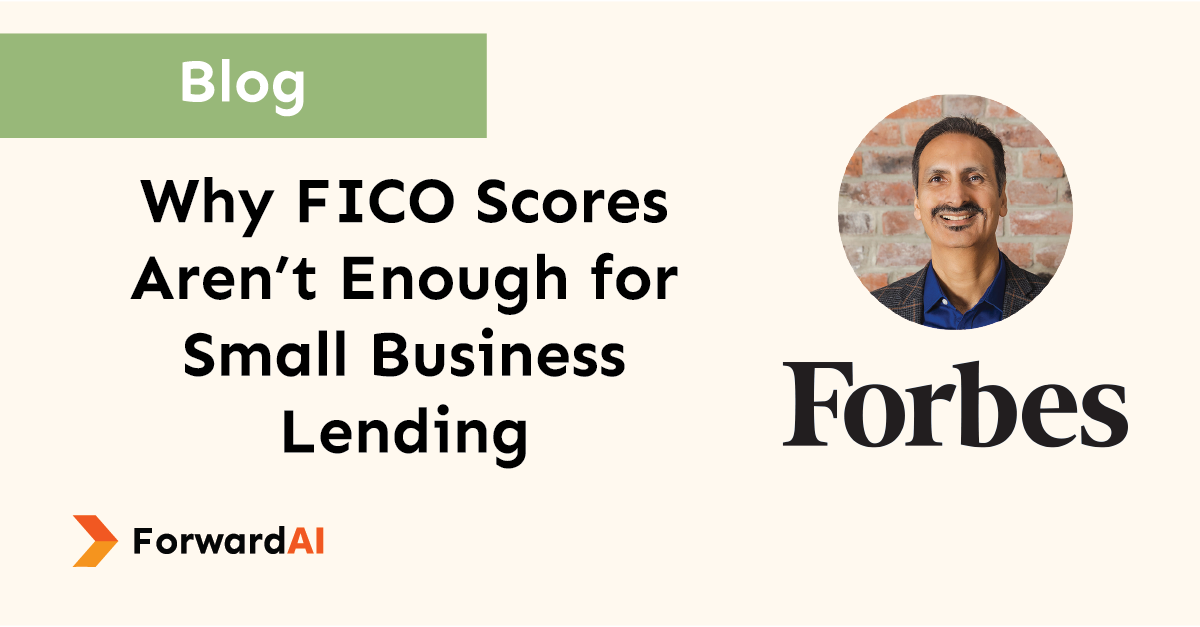
Originally shared on Forbes.com
By Nick Chandi – Forbes Councils Member
Nick Chandi is the CEO of ForwardAI, a market-leading provider of historical & forward-looking accounting data for small business lenders.
While there’s a growing demand for technology within almost every business sector, many fintech companies have been under more strain than ever since the Covid-19 pandemic caused economic uncertainty across the world. More people than ever spent 2020 looking for financial support, and many of them were owners of small businesses who found themselves having to lay themselves bare to potential lenders. Until recently, the FICO score was considered the holy grail of someone’s creditworthiness. It’s historically been the first metric that lenders consider. But post-pandemic, in a world where so many things have changed, is this still the case?
Since the pandemic began, I’ve helped hundreds of businesses get Paycheck Protection Program loans, Small Business Administration 7(a) loans and other short-term loans. I interact with forward-thinking lenders and financial institutions on an almost daily basis, and I find that they’re very passionate about helping small businesses as they’re the backbone of our economy.
Let’s consider where FICO scores came from. Originally designed to improve decisions using actionable historical data, by 1991, FICO scores were being accessed by the major credit bureaus. By 1995, they were being recommended for consideration in mortgage lending. FICO scores are based on five key components: payment history, current debt, length of credit history, new credit and types of credits. The higher a person’s FICO score, the less risky they’re typically considered to be.
Consider that component list in a post-pandemic world. How many businesses suddenly needed working capital or fell behind on payments while waiting for government assistance, even though there was a solid business plan to pivot and a healthy projected income stream?
While FICO has been updated over the years, it still falls short when it comes to providing current information about a potential borrower. There are simply too many issues with FICO, such as reporting errors that can hurt your score. Then there are people who don’t use credit cards and thus don’t have a score. Overall, a FICO score can’t tell you whether someone can meet a payment schedule. This leaves lenders with gaps to fill in with other documentation, which can slow down the underwriting workflow and open it up to errors as there’s so much data to manually parse.
It has quickly become overwhelmingly apparent that FICO is behind the times. But, as has been the case for three decades, this score is still considered a valuable piece of the underwriting process. So what can lenders do to fill in the cracks?
First things first, lenders can use a broader perspective to make their decisions. For instance, they can look to new types of data points created with innovative algorithms designed using machine learning. After all, predictive data has come a long way since “bugs” were just actual insects.
Some lenders already use some form of predictive data, such as a cash flow forecast, but in my experience with lenders, this work is usually completed manually. This is surprising when you consider how many cash flow forecasting programs are being adopted by small business owners and accountants. By automating their predictive data, lenders can cut down on data gathering and focus more on the actual decision-making.
The first step toward automation involves consumer-granted access to real-time data that lenders can use in their current cash flow templates. Getting direct permission from a borrower is the fastest way to automate. It allows lenders to access data directly instead of requesting it via document uploads, as 54% of lenders do for loans under $1 billion. Lenders can also consider partnering with one of the various companies that provide forecasting data to help expedite this process.
Cash flow data isn’t the only data that can be useful. With predictive algorithms fueled by artificial intelligence and machine learning, many more data points can be created to examine a borrower’s ability to pay. By projecting ratios and key performance indicators such as debt service coverage ratios, gross and net profit ratios, capital turnover rates, customer business trends and more, a lender can get a deep look into exactly who they’re lending to and how much they can afford. But this brings up another question: Is the accounting data accurate?
We all know about the concept of garbage in, garbage out. It’s regularly shared in any data-driven industry. When you’re dealing with something as easily manipulated as accounting data, it’s no different. Lenders need to take care to validate their borrowers’ accounting data, lest they make decisions based on ghost invoices.
One day soon, lenders across the world will be able to use automation technology to intake accounting and banking data and match the transactions to show just how accurate a borrower’s data is. A number of companies, including mine, are working on such technology.
In the meantime, financial institutions can still create predictive data manually. Using the “stare and compare” method to flip back and forth between PDFs, checking values, adding up numbers and typing it all into a template or a system, you can develop predictive data points. The challenge is that this can be a time-consuming, error-prone and staffing-heavy process even for the most prominent financial institutions.
Some lenders have already begun focusing on cash flow rather than FICO. However, so many still get so caught up in historical data review that they neglect to consider the most straightforward question: Will my borrower be able to afford this loan based on their cash flow? With predictive data points instead of just historical data, lenders can better picture what exactly their potential clients are capable of. They say a picture is worth a thousand words, but I think when a picture is made up of predictive data built using validated financial data, it’s worth far, far more than that.
Originally shared on Forbes.com